How to use this guide
This primer addresses the common questions that people have about artificial intelligence (AI) systems. It contains nontechnical explanations of common terms and concepts associated with AI and gives examples of how to write about AI systems without misleading people about their capabilities. Although this primer was originally designed primarily for journalists, we have found that it is a valuable resource for anyone communicating about AI.
For a list of resources on where to find technical experts, please see Finding Experts in AI. For information specifically on generative AI, please see our accompanying guide Intro to Generative AI. Visit Emerging Tech Primers to see all primers.
What is artificial intelligence?
Artificial intelligence (AI) has historically referred to a collection of technologies designed to emulate human intelligence. In recent years, the term has become synonymous with machine learning, a set of computer processes used to identify unintuitive patterns in data. Examples of AI today include speech recognition, autonomous vehicle navigation, and the generation of new content, such as text or images.
Although the words “artificial intelligence” may conjure scenes from science fiction, most tools labeled with the term “AI” are not the powerful thinking machines of Hollywood movies. “Artificial General Intelligence,” or AGI, is the widely-used term to refer to those not-yet-realized advanced technologies that could independently learn new capabilities. (See What is artificial general intelligence?) Historically, AI models were developed to accomplish specific individual tasks, but there are efforts to pursue AGI through combining some of these capabilities into “foundation models.” Today’s AI tools are more basic and already deeply embedded in a variety of sectors, including business, government, and even things as commonplace as the autofocus in your camera.
The definition of what counts as AI continues to evolve and remains a subject of debate. In fact, some take issue with the term “AI” altogether. (See Why don’t people like the term “artificial intelligence?”) Within this primer, “AI” will be used to refer to a collection of machine learning technologies that are designed to automate specific tasks.
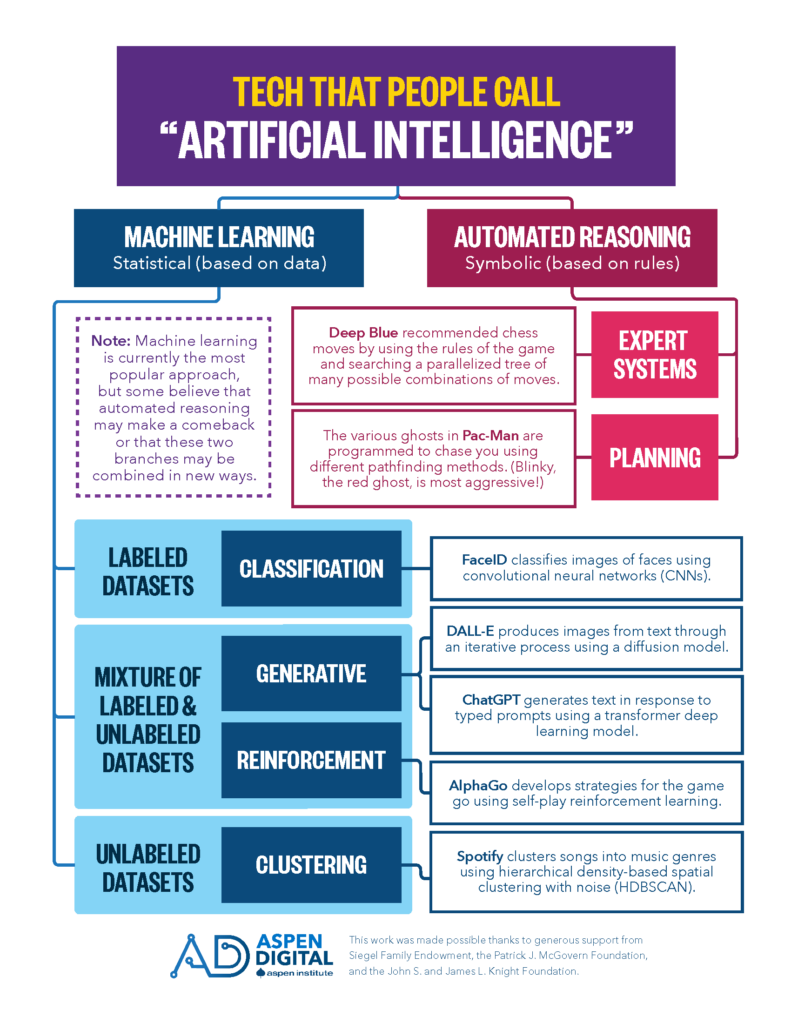
Throughout the years, there have been several technologies that people have called “Artificial Intelligence”
What is machine learning?
Machine learning systems are a type of AI that are essentially pattern recognition tools. They are “trained” to identify patterns within large collections of data (such as text, images, and video) in order to produce a set of instructions, or a “model,” which applies that “training” to new data. For instance, a machine learning model could be “trained” on news articles and then used to predict the next word in a sentence you are typing.
What is “the algorithm?”
Many people use the term “algorithm” colloquially to refer to a wide array of technologies (such as the Instagram algorithm, an encryption algorithm, or a facial recognition algorithm). Generally, the term “algorithm” is defined as a set of instructions for a computer to execute. However, when people talk about algorithms in relation to AI, they typically mean one of two related, but different, things:
1
The process of creating a model
When someone says a facial recognition system was created “by feeding images from Facebook into a machine learning algorithm,” that means that a machine learning model is being “trained” to represent the patterns in that image data to recognize faces.
2
The application of a model to produce an output
When someone says “the YouTube algorithm prefers short-form content,” they’re referring to how the model YouTube uses to recommend videos to watch next shows shorter videos to more people.
What is data, and why is it so important?
Data, like AI, is an umbrella term that covers more than just numbers. The term describes many types of information that are stored and processed on computers. Videos, electronic health records, and the location information on your phone are all different kinds of data.
More reliable AI systems typically require a large amount of data to “train.” This is because the patterns in a small collection of data may not be generalizable. For instance, a system built to label dogs in a collection of images may not operate reliably on images of dogs in a park if all of the examples used to “train” the system were of dogs in homes. Using more data from more diverse sources helps to ensure that the patterns represented in the machine learning model apply to a wide variety of contexts.
There is a tendency to misinterpret the accuracy of machine learning models as a measure of how well they represent reality. In fact, what academics and engineers often call the “accuracy” of AI systems is only a measure of how well they represent the data used to “train” them. If the data used to create a model is flawed (whether because it is incomplete or biased), the outputs of the model will reproduce or even amplify those flaws.
Non-exhaustive list of types of data
- Text (books, articles, blog posts, discussion threads, social media, health records)
- Images (photos, paintings and illustrations, x-rays, maps)
- Audio (voice, music, birdsong, engine noise)
- Video (CCTV, drone footage, film and television recordings)
- Biometrics (face, fingerprint, heart rhythm, gestures)
- Geolocation information
Having transparency into what data is used to “train” a model can give us insight into how the model responds to different examples. Generative AI systems like ChatGPT are trained on large swaths of the internet—knowing which parts of the internet are included in the training data makes ChatGPT’s output more explainable.
Why don’t people like the term “artificial intelligence?”
Many researchers and activists have argued that describing these systems as “intelligent” attributes too much agency to the technology itself and erases the humans involved in the process. When people talk about AI, they’ll often say things like “an AI fired 3000 workers” or “DALL-E created award-winning art.”
In reality, these tools—regardless of how much or little oversight they receive—do not exist in a vacuum. Humans choose what types of systems to develop and curate the data to “train” machine learning models. Humans define the criteria for good system performance, and humans deploy the resulting technology—even if they abdicate responsibility for its impacts. Critics argue that calling these tools “artificial intelligence” obfuscates the human roles in these processes and makes it difficult for people impacted by the deployment of AI to seek remedy or recourse. Although there are compelling arguments for abandoning terms like “artificial intelligence” and “machine learning,” they are nonetheless already in wide use. Rather than avoiding these terms, it may be more pragmatic to help the public contextualize them by both explaining what specific technologies constitute the AI systems being discussed and to highlight the people involved in building and using these tools. (See How to Talk About AI for examples.)
Learn more
What is artificial general intelligence?
Artificial general intelligence (AGI), sometimes referred to as “strong AI,” is a conceptual computational tool that exhibits human-level or beyond human-level intelligence in all domains. Some people believe it is important to pursue AGI because a machine that has generalizable, human-level intelligence could be a useful tool for space exploration, national security, or neuroscience. No such capabilities currently exist, though many companies are actively pursuing this possibility.
Throughout history, different tests have been proposed for identifying intelligence in AI systems, including playing chess, making a cup of coffee, or the “Turing test,” which, to pass, a human must fail to identify the AI in conversation. All of these have since been ruled insufficient indicators of human-like intelligence. Until the more philosophical question of how to define intelligence is addressed, there may be no agreed upon way to evaluate attempts to make these kinds of systems.
How to talk about A.I.
This section showcases examples of how to write about AI inspired by news stories from the last year. Reporting on AI should avoid personifying the technology or obfuscating the people and organizations using the tools. Instead, aim for sentences that clearly highlight both the types of technology being used and the people involved in their design and deployment. Researchers are often useful sources for getting more information on the specific capabilities and limitations of AI systems. (See Common Roles in AI for more information.)
In general, accurate descriptions include the following:
People who use specific AI tools or capabilities to do tasks.
examples
- Employers are automating data-entry and operational tasks by deploying natural language processing AI, which could put some administrative jobs at risk.
- People are using DALL-E to create art, which has professional illustrators worried about their futures.
- What has become apparent is that, even though large language models can be used to generate reasonable language, the models themselves lack the capacity to “know” what they are doing, and even experts are unsure of how the models perform as well as they do.
- The Post reported that local governments are deploying facial-recognition cameras to “scan everyone who walks past them,” looking for people who are banned from public housing.
- While these tools are not designed to be used for medical applications, patients are asking text-generators like ChatGPT to self-diagnose their medical conditions.
Acknowledgements
This work was produced by Eleanor Tursman, B Cavello, and Tom Latkowski, and was made possible thanks to generous support from Siegel Family Endowment, the Patrick J. McGovern Foundation, and the John S. and James L. Knight Foundation.
Share your Thoughts
If this work is helpful to you, please let us know! We actively solicit feedback on our work so that we can make it more useful to people like you.
Contact us with questions or corrections regarding this primer. Please note that Aspen Digital cannot guarantee access to experts or expert contact information, but we are happy to serve as a resource. To find experts, please refer to Finding Experts in AI.
AI 101 © 2023 by Aspen Digital is licensed under CC BY-NC 4.0.